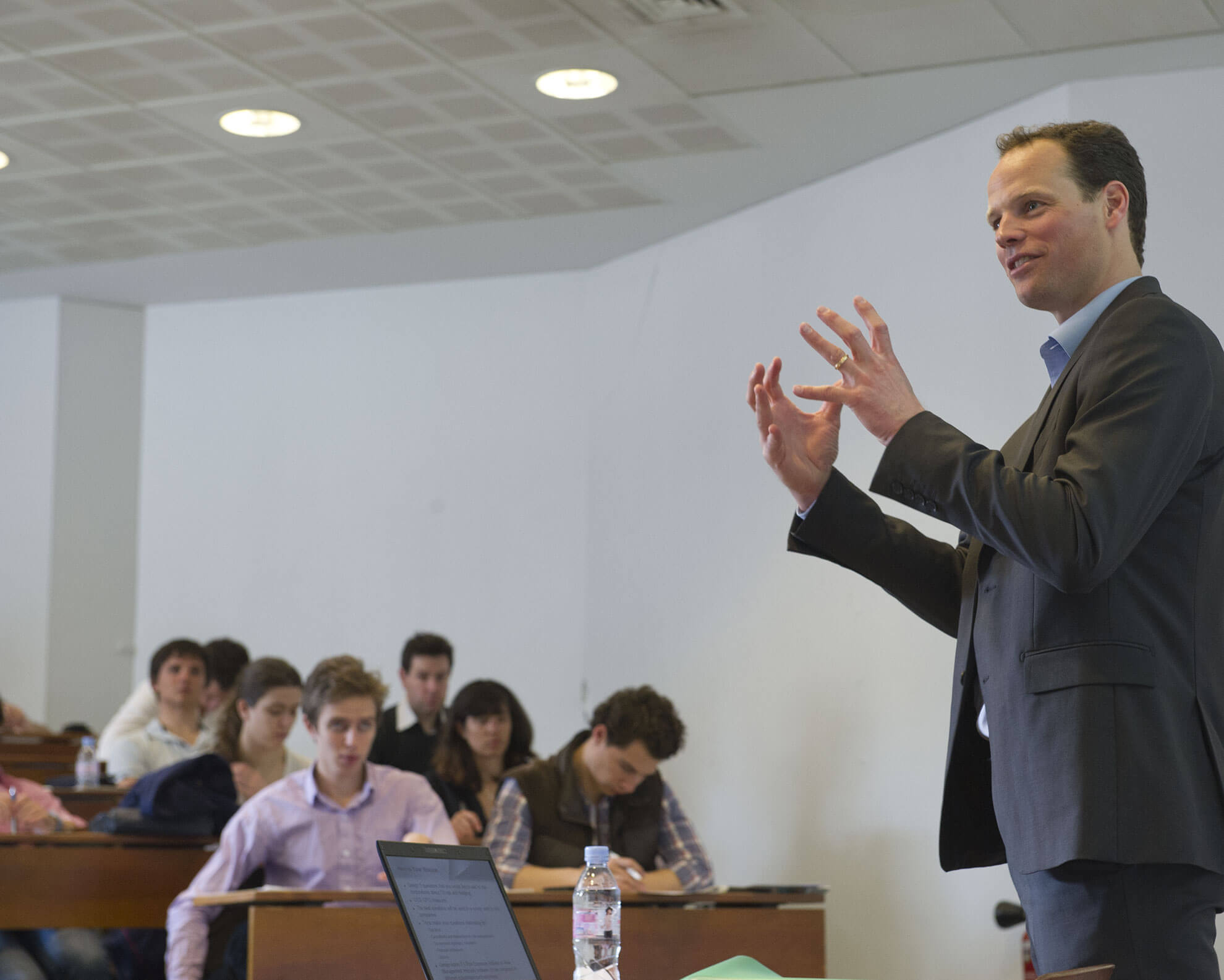
Faculty & Research
Faculty & Research Faculty & Research
HEC Paris is one of the world’s top research schools in economics and management. Our faculty push the boundaries of knowledge by producing the highest quality research. They aim to have an impact on pedagogy, management practices, public policies and society at large.
Research conducted at HEC Paris comprises both disciplinary and cross-disciplinary work, with a unique combination of scientific excellence and business relevance.
Events
Apr 30
2024
11:20 am
Jouy-En-Josas
English
Apr 30
2024
11:20 am
Jouy-En-Josas
English

Chairs
Endowed Chairs
Decision Sciences
AXA
Chair holder
Itzhak Gilboa
Professor, Economics and Decision Science Department
Endowed Chairs
Purposeful Leadership
Joly Family
Chair holder
Rodolphe Durand
Professor of Strategy and Academic Director of S&O Center
Endowed Chairs
Value Investing
Jean-Marie Eveillard
Augustin Landier
Professor of Finance, HEC Paris
Latest News
Key Facts
167
Full-time faculty
55
Ph.D. Students
33
Nationalities
> 15
Research Chairs & Corporate initiatives
4
Centers
45
articles published in the top academic reviews per year